Breaking News
US Army Rethinks and Revolutionizes Stryker Availability.
In a context where large-scale combat operations are becoming more frequent, the US Army recognizes the inadequacy of its current equipment availability prediction system. The traditional bank time model, limited in its ability to incorporate external factors such as training conditions, personnel strength, and parts availability, can only forecast equipment availability for a short period. Facing this challenge, the US Army is exploring more advanced analytical tools to improve its predictions.
Follow Army Recognition on Google News at this link
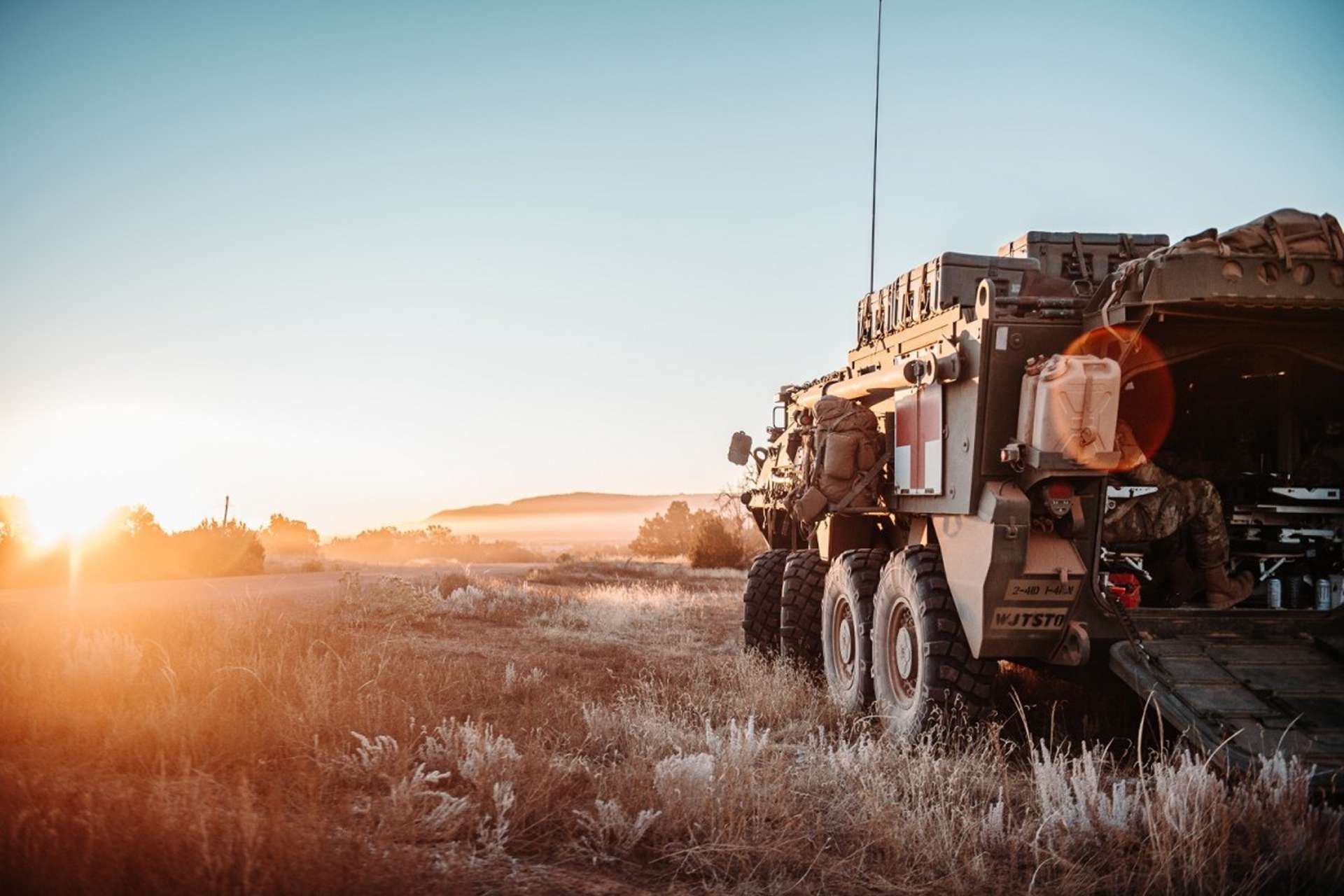
The Stryker is a US Armored personnel carrier. (Picture source: US DoD)
During a rotational deployment in Korea, the 2nd Stryker Brigade Combat Team, 4th Infantry Division, initiated an effort to integrate machine learning to improve equipment availability predictions, focusing particularly on the Stryker fleet of the 1st Battalion, 41st Infantry Regiment (1-41). Using training and maintenance data, the goal was to more accurately assess current and future equipment availability. This initiative demonstrated significant improvements in predictive accuracy through machine learning models based on time series data.
The team collected two years of daily maintenance and training data from a Stryker infantry battalion, beginning the analysis with linear regression. Although this model did not provide sufficient accuracy, it identified key variables influencing maintenance availability. Consequently, the team used more advanced machine learning tools and tested three models: Prophet, random-search/random-forest, and Bayesian gradient boosting regression. Prophet, an open-source model developed by Meta Platforms, showed the best results, outperforming traditional methods.
The initial data collection effort focused on an entire Stryker brigade but was scaled down to a single battalion to ensure training data consistency. Two datasets were used: one including days with no scheduled activity (DONSAs) and one excluding them. Maintenance data came from the Global Combat Support System-Army, and the battalion’s training schedules served as the exogenous dataset for forecasting.
The study revealed that completed services and DONSAs significantly impacted forecast accuracy. Removing the DONSA variable improved all models, highlighting the importance of adhering to service schedules for accurately predicting maintenance availability. The Prophet model, in particular, provides commanders with a clearer understanding of the impact of maintenance on future operations, allowing for better planning and resource allocation.
These models can also be used in large-scale combat operations to identify the units best positioned for specific missions, thereby improving decision-making and staff recommendations. Battalions can replicate these models using readily available daily data, leveraging soldiers' skills and existing tools like the Jupyter coding environment.
To maximize the benefits of these advancements, the Army must focus on three key areas: data availability, training, and software accessibility. By improving data interoperability and integrating data literacy into military education, the Army can enhance leaders' understanding of statistical models. Additionally, improving access to data transformation tools and collaborating with industry leaders like Palantir, AWS, and Microsoft will enable the Army to continue modernizing and using AI to improve military decision-making.
The "Stryker Ready" initiative represents a step towards integrating machine learning into military maintenance, demonstrating how available tools can transform operations and maximize the efficiency of the US Armed Forces.
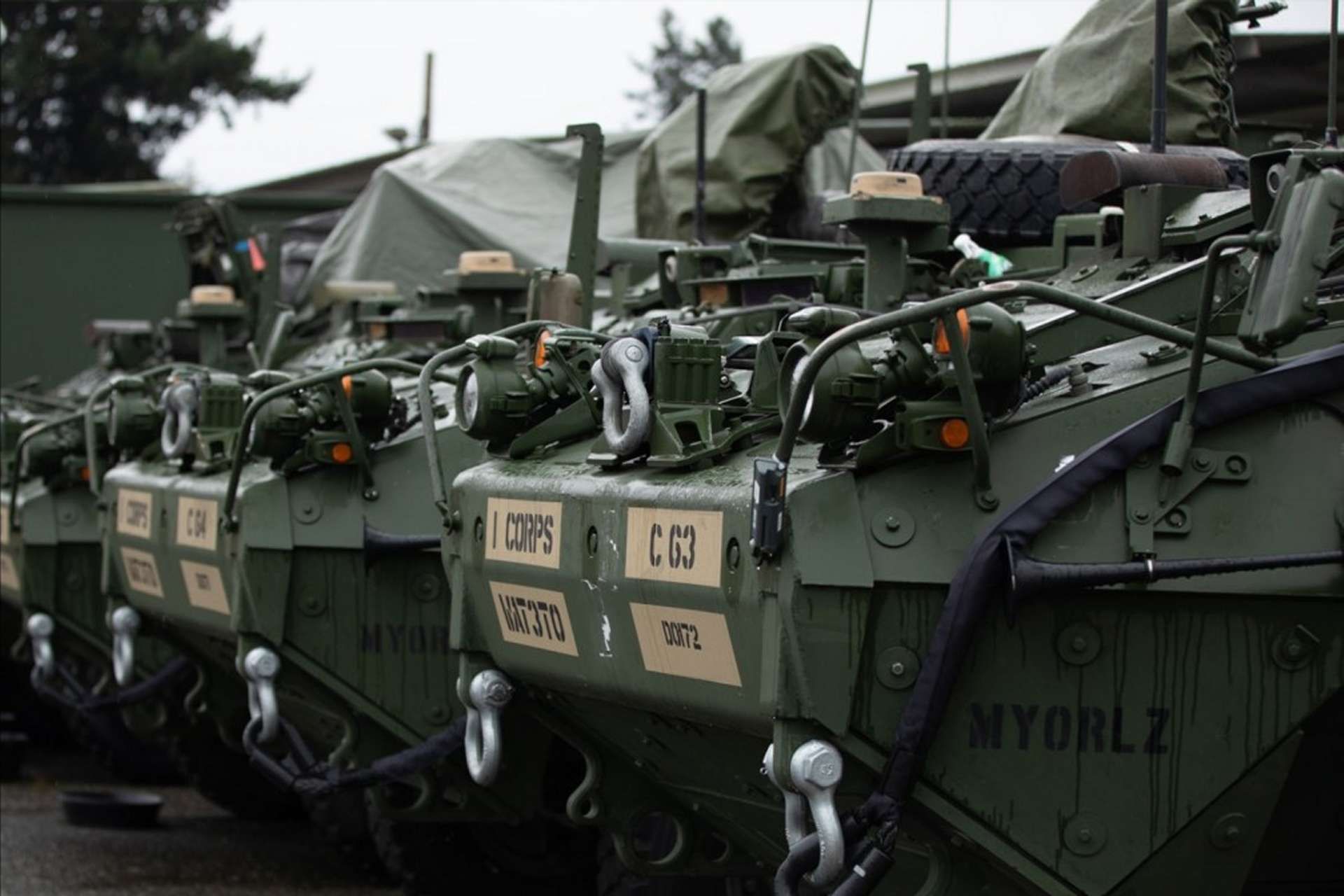
The US Army is exploring more advanced analytical tools to improve its Stryker Availability. (Picture source: US DoD)